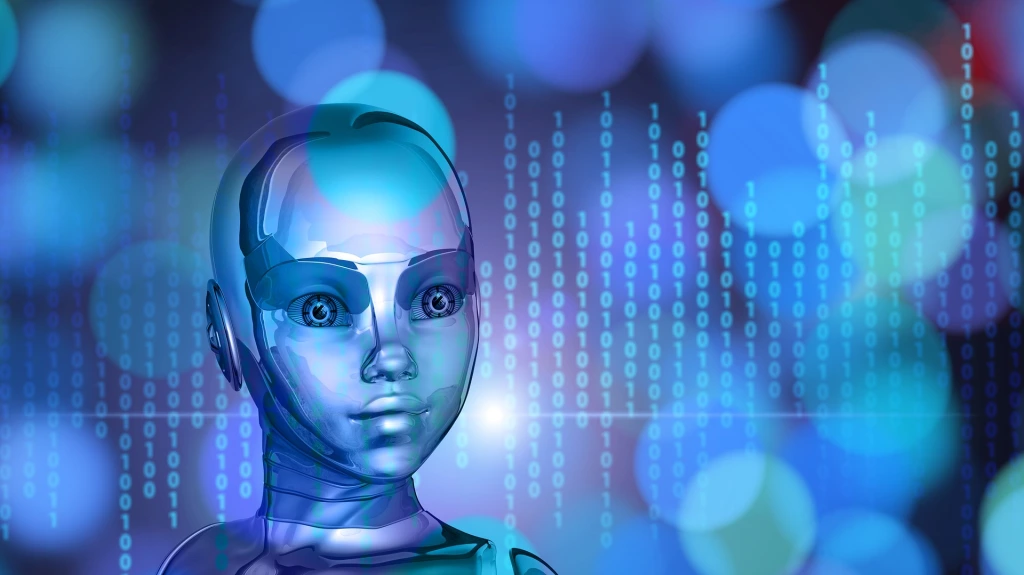
The drug R&D process is lengthy and expensive. The average cost of drug development is 2.6 billion USD and typically takes 10- 12 years, and can be broadly divided into four major stages:
- Target selection and validation.
- Compound screening and lead optimization.
- Preclinical studies.
- Clinical trials.
Majority of pharmaceutical companies face challenges in their drug development programs because of increased costs and reduced effectiveness of their research laboratories. Many discovery efforts go down the drain because 9 out of 10 candidate therapies fail between phase I trials and commercial/regulatory approval.
Applying AI/ML could shorten the R&D cycle and reduce the overall cost of bringing a drug to market. Overall, the way pharma discovers, develops drugs, and assesses them in trials comes down to sophisticated pattern recognition that can now be automated using AI. The application of AI/ML can help predict the mechanism of actions (MOA), clinical outcomes (safety/efficacy) of combined treatments by clustering public datasets, de-noising datasets, and bias detection. Algorithms can be trained on big data repositories such as public liberties cell culture, human and animal research models. Other sources as research papers, patents, clinical trials, and patient records can also be used. Insights are captured through data extraction, cleansing, validating, and outcome prediction of patient information. The massive data lakes can be formed through collaborations with other pharmaceutical companies to share data repositories and train algorithms.
AI use in drug discovery
Standard high-throughput screening library usually contains around 1 million compounds, where each compound typically costs 50–100 USD. Thus, an initial screening process can cost several million USD plus several months of work. Subsequent optimization of the lead compound might take several years to identify preclinical drug candidates.
By contrast, with AI’s help, a virtual compound library of several billion molecules can be screened within a few days. It might only take a few months to 1 year to identify preclinical candidates by using an AI-based computational pipeline. Traditional experimental structural biology methods usually take at least a few years to resolve a protein structure. AI-based time-efficient predicting of target proteins’ 3D structures takes few hours to a few days.
In general, the above processes are somewhat laborious to perform. With the help of AI, they can be automated and optimized to substantially speed up the drug discovery phase, thus shortening the pharmaceutical product’s overall R&D cycle. AI/ML has enormous potential to revolutionize drug discovery. Still, only large pharmaceutical companies experiment with AI technologies at this stage due to costs and operational complexities (e.g., talent pools necessary, such as data scientists and other AI experts).
Currently, computational methods, including AI, do not perform well in all drug research areas, and some aspects of the drug discovery process have not yet been well explored. Using AI/ML in pharmaceutical product development has its limitations due to a couple of reasons:
First, AI is a data-mining method, and the performance of AI models depends on the amount and quality of the available data. And successful training of DNNs (deep neural networks) relies on large amounts of training data. In the future, further development of transfer learning technology may allow the system to learn from one task and apply it to the other task and solve this problem.
Second, the quality of the available data is sometimes insufficient for efficient AI learning. Experimental data in public databases are often not measured with the same methods or conditions. Thus, different methods could yield different data that are not comparable with each other. Public databases may contain multiple, contradicting datasets. Therefore, before performing specific AI tasks, filtering the raw inputs for high-quality data is essential.
To conclude, a tremendous amount of work has been done to incorporate AI tools to expedite the drug discovery cycle, with some success, but resolving the problem of current AI imitations in drug R&D will be necessary before its full potential is realized.
Power of partnerships
There is a tremendous promise in ongoing and future partnerships in the AI space. A growing number of pharmaceutical companies invest both in internal AI-based R&D programs and in cooperation with AI startups and academic institutions. The number of those collaborations will continue increasing with time. The smaller companies, which have not jumped on the AI wagon yet, should start building the internal capacities for machine learning and expand partnerships with other pharmaceutical companies. It is the right step forward to maintain a competitive advantage, reduce R&D risks and cost and increase chances of product commercialization.
One way the companies collaborate is by applying AI partnerships in federate learning. According to Wikipedia, federated learning (also known as collaborative learning) is a machine learning technique that trains an algorithm across multiple decentralized edge devices or servers holding local data samples without exchanging them. Local algorithms are trained on local data samples, and the weights (the learnable parameters of the algorithms) are exchanged between the algorithms at some frequency to generate a global model. They train their predictive models in a de-identified, aggregated fashion without exposing private research, data, or information.
As of today, there are no approved AI-developed drugs. The hype can’t last very long because the truth will come out in the data over 3-5 years. We will learn if pharmaceutical companies, that experiment with AI/ML in drug R&D, do it faster and cheaper. If yes, AI will take off and separate the winners and early adopters from losers (late adopters).